Fill in Blanks
Home
1.1 Bivariate Relationships
1.2 Probabilistic Models
1.3 Estimation of the Line
1.4 Properties of the Least Squares Estimators
1.5 Estimation of the Variance
2.1 The Normal Errors Model
2.2 Inferences for the Slope
2.3 Inferences for the Intercept
2.4 Correlation and Coefficient of Determination
2.5 Estimating the Mean Response
2.6 Predicting the Response
3.1 Residual Diagnostics
3.2 The Linearity Assumption
3.3 Homogeneity of Variance
3.4 Checking for Outliers
3.5 Correlated Error Terms
3.6 Normality of the Residuals
4.1 More Than One Predictor Variable
4.2 Estimating the Multiple Regression Model
4.3 A Primer on Matrices
4.4 The Regression Model in Matrix Terms
4.5 Least Squares and Inferences Using Matrices
4.6 ANOVA and Adjusted Coefficient of Determination
4.7 Estimation and Prediction of the Response
5.1 Multicollinearity and Its Effects
5.2 Adding a Predictor Variable
5.3 Outliers and Influential Cases
5.4 Residual Diagnostics
5.5 Remedial Measures
4.6 ANOVA and Adjusted Coefficient of Determination
"I can prove anything by statistics except the truth." - George Canning
Recall from Figure 2.4.2
in Section 2.4.3 that we used $SS_{YY}$ to denote the variability of the response variable $Y$ from its mean $\bar Y$ (without regard to the model involving $X$).
Another name for $SS_{YY}$ is thesum of squares total (SSTO). We call it this since it gives us a measure of total variability in $Y$.
In multiple regression, SSTO is still the same as $SS_{YY}$ in (2.12)
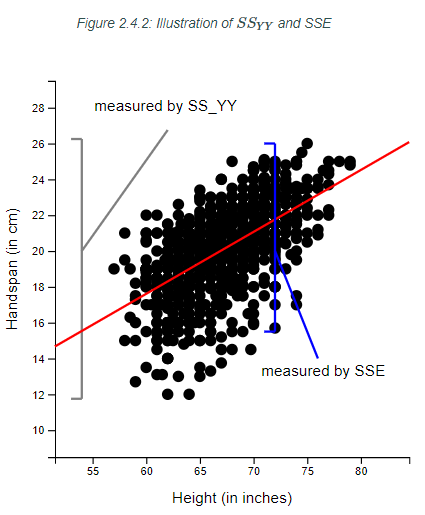
Another name for $SS_{YY}$ is the
In multiple regression, SSTO is still the same as $SS_{YY}$ in (2.12)
$$
SS_{YY} = \sum\left(Y_i-\bar{Y}\right)^2\qquad\qquad\qquad(2.12)
$$
. In matrix notation this can be expressed as
\begin{align*}
SSTO & ={\bf Y}^{\prime}{\bf Y}-\left(\frac{1}{n}\right){\bf Y}^{\prime}{\bf J}{\bf Y}\qquad(4.34)
\end{align*}
Also recall from Figure 2.4.2
, the variability of $Y$ about the regression line (in simple linear regression) was expressed by SSE in (1.16)
In multiple regression, SSE is still the sum of the square distances between the response $Y$ and the fitted model $\hat{Y}$. Now, the fitted model is the fitted hyperplane instead of a line.
The SSE can be expressed in matrix terms as \begin{align*} SSE & =\left({\bf Y}-{\bf X}{\bf b}\right)^{\prime}\left({\bf Y}-{\bf X}{\bf b}\right)\\ & ={\bf Y}^{\prime}{\bf Y}-{\bf b}^{\prime}{\bf X}^{\prime}{\bf Y}\qquad\qquad\left(4.35\right) \end{align*}
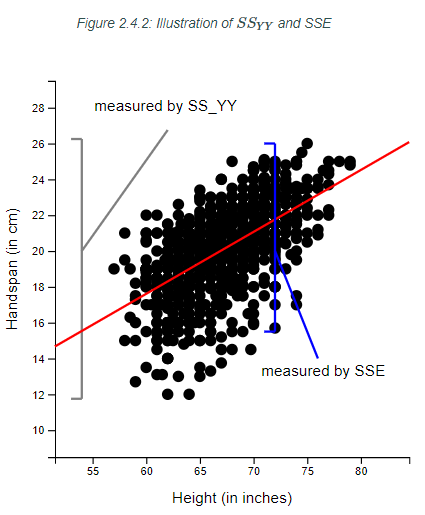
$$
SSE = \sum \left(Y_i - \hat{Y}_i\right)^2\qquad\qquad\qquad(1.16)
$$
. We can think of this as the variability of $Y$ remaining after exampling some of the variability with the regression model.
In multiple regression, SSE is still the sum of the square distances between the response $Y$ and the fitted model $\hat{Y}$. Now, the fitted model is the fitted hyperplane instead of a line.
The SSE can be expressed in matrix terms as \begin{align*} SSE & =\left({\bf Y}-{\bf X}{\bf b}\right)^{\prime}\left({\bf Y}-{\bf X}{\bf b}\right)\\ & ={\bf Y}^{\prime}{\bf Y}-{\bf b}^{\prime}{\bf X}^{\prime}{\bf Y}\qquad\qquad\left(4.35\right) \end{align*}
If SSTO is the total variability of $Y$ (without of regard to the
predictor variables), and SSE is the variability of $Y$ left over
after explaining the variability of $Y$ with the model (including the predictor variables), we might want
to know the variability of $Y$ explained by the model.
We call the variability explained by the regression model thesum of squares regression (SSR) .
We will show below that SSR can be expressed as \begin{align*} SSR & =\sum\left(\hat{Y}_{i}-\bar{Y}\right)^{2}\qquad(4.36) \end{align*} which can be expressed in matrix terms as \begin{align*} SSR & ={\bf b}^{\prime}{\bf X}^{\prime}{\bf Y}-\left(\frac{1}{n}\right){\bf Y}^{\prime}{\bf J}{\bf Y}\qquad(4.37) \end{align*}
We call the variability explained by the regression model the
We will show below that SSR can be expressed as \begin{align*} SSR & =\sum\left(\hat{Y}_{i}-\bar{Y}\right)^{2}\qquad(4.36) \end{align*} which can be expressed in matrix terms as \begin{align*} SSR & ={\bf b}^{\prime}{\bf X}^{\prime}{\bf Y}-\left(\frac{1}{n}\right){\bf Y}^{\prime}{\bf J}{\bf Y}\qquad(4.37) \end{align*}
To see how SSTO, SSR, and SSE relate to each other, consider how SSTO
is a sum of squares of $Y$ from its mean $\bar{Y}$:
\begin{align*}
Y_{i}-\bar{Y}
\end{align*}
We can add and subtract the fitted value $\hat{Y}_{i}$ to get
\begin{align*}
Y_{i}-\bar{Y} & =Y_{i}-\hat{Y}_{i}+\hat{Y}_{i}-\bar{Y}\\
& =\left(Y_{i}-\hat{Y}_{i}\right)+\left(\hat{Y}_{i}-\bar{Y}\right)
\end{align*}
Squaring both sides gives us
\begin{align*}
\left(Y_{i}-\bar{Y}\right)^{2} & =\left[\left(Y_{i}-\hat{Y}_{i}\right)+\left(\hat{Y}_{i}-\bar{Y}\right)\right]^{2}\\
& =\left(Y_{i}-\hat{Y}_{i}\right)^{2}+\left(\hat{Y}_{i}-\bar{Y}\right)^{2}+2\left(Y_{i}-\hat{Y}_{i}\right)\left(\hat{Y}_{i}-\bar{Y}\right)
\end{align*}
Summing both sides gives us
\begin{align*}
\sum\left(Y_{i}-\bar{Y}\right)^{2} & =\sum\left(Y_{i}-\hat{Y}_{i}\right)^{2}+\sum\left(\hat{Y}_{i}-\bar{Y}\right)^{2}+2\sum\left(Y_{i}-\hat{Y}_{i}\right)\left(\hat{Y}_{i}-\bar{Y}\right)\\
& =\sum\left(Y_{i}-\hat{Y}_{i}\right)^{2}+\sum\left(\hat{Y}_{i}-\bar{Y}\right)^{2}+2\sum\hat{Y}_{i}e_{i}-2\bar{Y}\sum e_{i}
\end{align*}
Note that $\sum\hat{Y}_{i}e_{i}=0$ from (1.22)
decomposition of SSTO.
\begin{align*}
\sum\hat{Y}_{i}e_{i} & =0 & \qquad\qquad\qquad(1.22)
\end{align*}
and $\sum e_{i}=0$
from (1.20)
\begin{align*}
\sum e_{i} & =0 & \qquad\qquad\qquad(1.20)
\end{align*}
.
Therefore, we have
\begin{align*}
\sum\left(Y_{i}-\bar{Y}\right)^{2} & =\sum\left(\hat{Y}_{i}-\bar{Y}\right)^{2}+\sum\left(Y_{i}-\hat{Y}_{i}\right)^{2}\\
SSTO & =SSR+SSE \qquad\qquad\qquad\qquad\qquad(4.38)
\end{align*}
We call this the
The degrees of freedom can be decomposed as well. Note that the degrees
of freedom for SSTO is
\begin{align*}
df_{SSTO} & =n-1
\end{align*}
since the mean of $Y$ is needed to be estimated with $\bar{Y}$.
The degrees of freedom for SSE is
\begin{align*}
df_{SSE} & =n-p
\end{align*}
since the $p$ coefficients $\beta_{0},\ldots,\beta_{p-1}$ need
to be estimated with $b_{0},\ldots,b_{p-1}.$
For SSR, the degrees of freedom is
\begin{align*}
df_{SSR} & =p-1
\end{align*}
since there are $p$ estimated coefficients $b_{0},\ldots,b_{p-1}$
but need to estimate the mean of $Y$ with $\bar{Y}$.
Decomposing the degrees of freedom give us
\begin{align*}
n-1 & =p-1+n-p\\
df_{SSTO} & =df_{SSR}+df_{SSE}\qquad(4.29)
\end{align*}
The sums of squares and degrees of freedom are commonly displayed
in an analysis of variance (ANOVA) table:
Source | df | SS | MS | F | p-value |
---|---|---|---|---|---|
Regression | $df_{SSR}$ | $SSR$ | |||
Error | $df_{SSE}$ | $SSE$ | |||
Total | $df_{SSTO}$ | $SSTO$ |
Recall from Section 3.1.1 that if we divide SSE by its degrees of
freedom, we obtain the mean square error:
\begin{align*}
MSE & =\frac{SSE}{n-p}\qquad(4.40)
\end{align*}
Likewise, if we divide SSR by its degrees of freedom, we obtain the
mean square regression :
\begin{align*}
MSR & =\frac{SSR}{p-1}\qquad(4.41)
\end{align*}
These values are also included in the ANOVA table:
Note that although the sum of squares and degrees of freedom decompose, the mean squares do not. That is \begin{align*} \frac{SSTO}{n-1} & \ne MSR+MSE \end{align*} In fact, the mean square for the total ($SSTO/n-1$) does not usually show up on the ANOVA table.
Source | df | SS | MS | F | p-value |
---|---|---|---|---|---|
Regression | $df_{SSR}$ | $SSR$ | $MSR$ | ||
Error | $df_{SSE}$ | $SSE$ | $MSE$ | ||
Total | $df_{SSTO}$ | $SSTO$ |
Note that although the sum of squares and degrees of freedom decompose, the mean squares do not. That is \begin{align*} \frac{SSTO}{n-1} & \ne MSR+MSE \end{align*} In fact, the mean square for the total ($SSTO/n-1$) does not usually show up on the ANOVA table.
In Section 2.2.4, we tested the slope in simple regression to see
if there is a significant linear relationship between $X$ and $Y$.
In multiple regression, we will want to see if there is any significant linear relationship between any of the $X$s and $Y$. Thus, we want to test the hypotheses \begin{align*} H_{0}: & \beta_{1}=\beta_{2}=\cdots=\beta_{p-1}=0\\ H_{a}: & \text{at least one } \beta \text{ is not equal to zero} \end{align*} To construct a test statistic, we first note that \begin{align*} \frac{SSE}{\sigma^{2}} & \sim\chi^{2}\left(n-p\right) \end{align*} Also, if $H_{0}$ is true, then \begin{align*} \frac{SSR}{\sigma^{2}} & \sim\chi^{2}\left(p-1\right) \end{align*} The ratio of two independent chi-square random variables divided by their degrees of freedom give a statistic that follows a F-distribution.
Since $SSE/\sigma^{2}$ and $SSR/\sigma^{2}$ are independent (proof not give here), then under $H_{0}$, we can construct a test statistic as \begin{align*} F^{*} & =\left(\frac{\frac{SSR}{\sigma^{2}}}{p-1}\right)\div\left(\frac{\frac{SSE}{\sigma^{2}}}{n-p}\right)\\ & =\left(\frac{SSR}{p-1}\right)\div\left(\frac{SSE}{n-p}\right)\\ & =\frac{MSR}{MSE}\qquad\qquad\qquad\qquad(4.42) \end{align*} Large values of $F^{*}$ indicate evidence for $H_{a}$.
The test statistic and p-value are the last two components of the ANOVA table:
In multiple regression, we will want to see if there is any significant linear relationship between any of the $X$s and $Y$. Thus, we want to test the hypotheses \begin{align*} H_{0}: & \beta_{1}=\beta_{2}=\cdots=\beta_{p-1}=0\\ H_{a}: & \text{at least one } \beta \text{ is not equal to zero} \end{align*} To construct a test statistic, we first note that \begin{align*} \frac{SSE}{\sigma^{2}} & \sim\chi^{2}\left(n-p\right) \end{align*} Also, if $H_{0}$ is true, then \begin{align*} \frac{SSR}{\sigma^{2}} & \sim\chi^{2}\left(p-1\right) \end{align*} The ratio of two independent chi-square random variables divided by their degrees of freedom give a statistic that follows a F-distribution.
Since $SSE/\sigma^{2}$ and $SSR/\sigma^{2}$ are independent (proof not give here), then under $H_{0}$, we can construct a test statistic as \begin{align*} F^{*} & =\left(\frac{\frac{SSR}{\sigma^{2}}}{p-1}\right)\div\left(\frac{\frac{SSE}{\sigma^{2}}}{n-p}\right)\\ & =\left(\frac{SSR}{p-1}\right)\div\left(\frac{SSE}{n-p}\right)\\ & =\frac{MSR}{MSE}\qquad\qquad\qquad\qquad(4.42) \end{align*} Large values of $F^{*}$ indicate evidence for $H_{a}$.
The test statistic and p-value are the last two components of the ANOVA table:
Source | df | SS | MS | F | p-value |
---|---|---|---|---|---|
Regression | $df_{SSR}$ | $SSR$ | $MSR$ | $F^*$ | $P\left(Z\ge Z^*\right)$ |
Error | $df_{SSE}$ | $SSE$ | $MSE$ | ||
Total | $df_{SSTO}$ | $SSTO$ |
As was the case with the simple regression model, the coefficient of determination for the multiple regression model (or the coefficient of multiple determination ) is
\begin{align*}
R^{2} & =\frac{SSR}{SSTO}\\
& =1-\frac{SSE}{SSTO}\qquad(4.43)
\end{align*}
The interpretation is still the same: it gives the proportion of the variation in $Y$ explained by the model using the predictor variables.
It is of importance to note that $R^{2}$ cannot decrease when adding
another $X$ to the model. It can either increase (if the new $X$
explains more of the variability of $Y$) or stay the same (if the
new $X$ does not explain more of the variability of $Y$). This can
be seen in (4.43) by noting that SSE cannot become larger by including
more $X$ variables and SSTO stays the same regardless of which $X$
variables are used.
Because of this, $R^{2}$ cannot be used for comparing the fit of models with different subsets of the $X$ variables.
A modified version of the $R^{2}$ could be used that adjusts for the number of $X$ variables. It is called theadjusted coefficient
of determination denoted as $R_{a}^{2}$.
In $R_{a}^{2}$, SSE and SSTO are divided by their respective degrees of freedom: \begin{align*} R_{a}^{2} & =1-\frac{\frac{SSE}{n-p}}{\frac{SSTO}{n-1}}\\ & =1-\left(\frac{n-1}{n-p}\right)\frac{SSE}{SSTO}\qquad(4.44) \end{align*} The value of $R_{a}^{2}$ can decrease when another $X$ is included in the model because any decrease in SSE may be more than offset by the loss of a degree of freedom of SSE ($n-p$).
Because of this, $R^{2}$ cannot be used for comparing the fit of models with different subsets of the $X$ variables.
A modified version of the $R^{2}$ could be used that adjusts for the number of $X$ variables. It is called the
In $R_{a}^{2}$, SSE and SSTO are divided by their respective degrees of freedom: \begin{align*} R_{a}^{2} & =1-\frac{\frac{SSE}{n-p}}{\frac{SSTO}{n-1}}\\ & =1-\left(\frac{n-1}{n-p}\right)\frac{SSE}{SSTO}\qquad(4.44) \end{align*} The value of $R_{a}^{2}$ can decrease when another $X$ is included in the model because any decrease in SSE may be more than offset by the loss of a degree of freedom of SSE ($n-p$).
Let's look at the bodyfat data from Example 4.5.1.
library(tidyverse)
dat = read.table("http://www.jpstats.org/Regression/data/BodyFat.txt", header=T)
fit = lm(bfat~tri+thigh+midarm, data=dat)
# we can get the multiple R^2 and adjusted R^2 from the summary
fit %>% summary
Call:
lm(formula = bfat ~ tri + thigh + midarm, data = dat)
Residuals:
Min 1Q Median 3Q Max
-3.7263 -1.6111 0.3923 1.4656 4.1277
Coefficients:
Estimate Std. Error t value Pr(>|t|)
(Intercept) 117.085 99.782 1.173 0.258
tri 4.334 3.016 1.437 0.170
thigh -2.857 2.582 -1.106 0.285
midarm -2.186 1.595 -1.370 0.190
Residual standard error: 2.48 on 16 degrees of freedom
Multiple R-squared: 0.8014, Adjusted R-squared: 0.7641
F-statistic: 21.52 on 3 and 16 DF, p-value: 7.343e-06
# to get them individually
summary(fit)$r.squared
[1] 0.8013586
summary(fit)$adj.r.squared
[1] 0.7641133
# The F test statistic and p-value can also be found from summary
summary(fit)
Call:
lm(formula = bfat ~ tri + thigh + midarm, data = dat)
Residuals:
Min 1Q Median 3Q Max
-3.7263 -1.6111 0.3923 1.4656 4.1277
Coefficients:
Estimate Std. Error t value Pr(>|t|)
(Intercept) 117.085 99.782 1.173 0.258
tri 4.334 3.016 1.437 0.170
thigh -2.857 2.582 -1.106 0.285
midarm -2.186 1.595 -1.370 0.190
Residual standard error: 2.48 on 16 degrees of freedom
Multiple R-squared: 0.8014, Adjusted R-squared: 0.7641
F-statistic: 21.52 on 3 and 16 DF, p-value: 7.343e-06
#you can obtain a version of the anova table:
anova(fit)
Analysis of Variance Table
Response: bfat
Df Sum Sq Mean Sq F value Pr(>F)
tri 1 352.27 352.27 57.2768 1.131e-06 ***
thigh 1 33.17 33.17 5.3931 0.03373 *
midarm 1 11.55 11.55 1.8773 0.18956
Residuals 16 98.40 6.15
---
Signif. codes: 0 ‘***’ 0.001 ‘**’ 0.01 ‘*’ 0.05 ‘.’ 0.1 ‘ ’ 1
#note that the Df, sum Sq, Mean Sq, and F test are split among the
#X variables