Fill in Blanks
Home
1.1 Bivariate Relationships
1.2 Probabilistic Models
1.3 Estimation of the Line
1.4 Properties of the Least Squares Estimators
1.5 Estimation of the Variance
2.1 The Normal Errors Model
2.2 Inferences for the Slope
2.3 Inferences for the Intercept
2.4 Correlation and Coefficient of Determination
2.5 Estimating the Mean Response
2.6 Predicting the Response
3.1 Residual Diagnostics
3.2 The Linearity Assumption
3.3 Homogeneity of Variance
3.4 Checking for Outliers
3.5 Correlated Error Terms
3.6 Normality of the Residuals
4.1 More Than One Predictor Variable
4.2 Estimating the Multiple Regression Model
4.3 A Primer on Matrices
4.4 The Regression Model in Matrix Terms
4.5 Least Squares and Inferences Using Matrices
4.6 ANOVA and Adjusted Coefficient of Determination
4.7 Estimation and Prediction of the Response
5.1 Multicollinearity and Its Effects
5.2 Adding a Predictor Variable
5.3 Outliers and Influential Cases
5.4 Residual Diagnostics
5.5 Remedial Measures
2.3 Inferences for the Intercept
"I think Comic Sans always screams FUN."
- Jerry Gergich (Parks and Recreation)
The interpretation for the intercept $\beta_0$ in model (2.1)
In Sections 2.5 and 2.6, we will discuss how to use the model for estimation and prediction. In these inferences, the estimation or prediction should only be made in the range of the values of $X$. This is because the information we have is only on that range. Therefore, we should only make inferences on what we have information for.
In Figure 2.3.1, a scatterplot of engine displacement vs mile per gallon (mpg) are shown for 32 vehicles. This data is themtcars dataset in the datasets library.
Suppose the red points in the plot are the only observations we have. We fit model (2.1)
Now suppose we use the fitted model to predict the mpg of a vehicle with an engine with a displacement of 258. The black point on the line at $X=258$ is the predicted mpg of 7.079638. Clearly, this value of displacement is beyond any displacement that we used to fit the model.
Consider the gray points as observations that we did not have to fit the model. Note how the regression line does not model those gray points very well. In fact, our prediction of 7.079638 mpg is far below an actual observation of an engine with displacement of 159 which had a mpg of 21.4.
This example shows that we should not use the model outside the range of the values of $X$. To do so is calledextrapolating and forces us to make assumptions about the relationship between $X$ and $Y$ that we have no information for.
We should also avoid making an interpretation of the intercept if the range of $X$ does not contain zero. So we should only interpret $\beta_0$ as the mean value of $Y$ when $X=0$ if zero is in the range of $X$.
\begin{align*}
Y_i=&\beta_0+\beta_1X_i+\varepsilon_i\\
\varepsilon_i\overset{iid}{\sim}& N\left(0,\sigma^2\right)\qquad\qquad\qquad\qquad(2.1)
\end{align*}
may not be useful for many applications.
In Sections 2.5 and 2.6, we will discuss how to use the model for estimation and prediction. In these inferences, the estimation or prediction should only be made in the range of the values of $X$. This is because the information we have is only on that range. Therefore, we should only make inferences on what we have information for.
In Figure 2.3.1, a scatterplot of engine displacement vs mile per gallon (mpg) are shown for 32 vehicles. This data is the
Suppose the red points in the plot are the only observations we have. We fit model (2.1)
\begin{align*}
Y_i=&\beta_0+\beta_1X_i+\varepsilon_i\\
\varepsilon_i\overset{iid}{\sim}& N\left(0,\sigma^2\right)\qquad\qquad\qquad\qquad(2.1)
\end{align*}
to only those red points. The blue line is the resulting least squares line.
Figure 2.3.1: Scatterplot of mtcars data
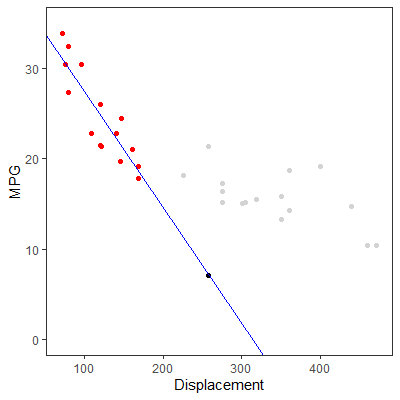
Consider the gray points as observations that we did not have to fit the model. Note how the regression line does not model those gray points very well. In fact, our prediction of 7.079638 mpg is far below an actual observation of an engine with displacement of 159 which had a mpg of 21.4.
This example shows that we should not use the model outside the range of the values of $X$. To do so is called
We should also avoid making an interpretation of the intercept if the range of $X$ does not contain zero. So we should only interpret $\beta_0$ as the mean value of $Y$ when $X=0$ if zero is in the range of $X$.
The BLUE for $\beta_{0}$ is the least squares estimator $b_0$.
In Section 1.4.2 and Section 1.4.3 we discussed that the mean of the sampling distribution of $b_{0}$ is $$ E[b_0]=\beta_0 $$ with a variance of \begin{align*} Var\left[b_{0}\right] & =\sigma^{2}\left[\frac{1}{n}+\frac{\left(\bar{X}\right)^{2}}{\sum \left(X_{i}-\bar{X}\right)^{2}}\right]\qquad\qquad\qquad(1.15) \end{align*}
As we did with $b_1$, we will assume the normal errors model (2.1)
Recall that we can write $b_{0}$ as \begin{align*} b_{0} & =\sum c_{i}Y_{i}\qquad\qquad\qquad(1.6) \end{align*} where \begin{align*} c_{i} & =\frac{1}{n}-\bar{X}k_{i} \end{align*} which shows that $b_{0}$ is alinear combination of $Y$.
Since $Y$ is normally distributed by (2.2)
In Section 1.4.2 and Section 1.4.3 we discussed that the mean of the sampling distribution of $b_{0}$ is $$ E[b_0]=\beta_0 $$ with a variance of \begin{align*} Var\left[b_{0}\right] & =\sigma^{2}\left[\frac{1}{n}+\frac{\left(\bar{X}\right)^{2}}{\sum \left(X_{i}-\bar{X}\right)^{2}}\right]\qquad\qquad\qquad(1.15) \end{align*}
As we did with $b_1$, we will assume the normal errors model (2.1)
\begin{align*}
Y_i=&\beta_0+\beta_1X_i+\varepsilon_i\\
\varepsilon_i\overset{iid}{\sim}& N\left(0,\sigma^2\right)\qquad\qquad\qquad\qquad(2.1)
\end{align*}
and examine the sampling distribution.
Recall that we can write $b_{0}$ as \begin{align*} b_{0} & =\sum c_{i}Y_{i}\qquad\qquad\qquad(1.6) \end{align*} where \begin{align*} c_{i} & =\frac{1}{n}-\bar{X}k_{i} \end{align*} which shows that $b_{0}$ is a
Since $Y$ is normally distributed by (2.2)
\begin{align*}
Y_i{\sim} N\left(\beta_0+\beta_1X_i,\sigma^2\right)\qquad\qquad\qquad(2.2)
\end{align*}
, then we can apply Theorem 2.1
\begin{align*}
Y_i=&\beta_0+\beta_1X_i+\varepsilon_i\\
\varepsilon_i\overset{iid}{\sim}& N\left(0,\sigma^2\right)\qquad\qquad\qquad\qquad(2.1)
\end{align*}
which
implies that $b_{0}$ is normally distributed. That is,
\begin{align*}
b_{0} & \sim N\left(\beta_{0},\sigma^2\left[\frac{1}{n}+\frac{\left(\bar{X}\right)^2}{\sum \left(X_i - \bar{X}\right)^2}\right]\right)\quad\quad\quad(2.7)
\end{align*}
Since $b_{0}$ is normally distributed, we can standardize it so that
the resulting statistic will have a standard normal distribution.
Therefore, we have \begin{align*} z=\frac{b_{0}-\beta_{0}}{\sqrt{\sigma^2\left[\frac{1}{n}+\frac{\left(\bar{X}\right)^2}{\sum \left(X_i - \bar{X}\right)^2}\right]}} & \sim N\left(0,1\right)\qquad\qquad\qquad(2.8) \end{align*}
Therefore, we have \begin{align*} z=\frac{b_{0}-\beta_{0}}{\sqrt{\sigma^2\left[\frac{1}{n}+\frac{\left(\bar{X}\right)^2}{\sum \left(X_i - \bar{X}\right)^2}\right]}} & \sim N\left(0,1\right)\qquad\qquad\qquad(2.8) \end{align*}
As we did with $b_1$ in Section 2.2.2, we can studentized $b_0$ to obtain
\begin{align*}
t & =\frac{b_{0}-\beta_{0}}{\sqrt{s^2\left[\frac{1}{n}+\frac{\left(\bar{X}\right)^2}{\sum \left(X_i - \bar{X}\right)^2}\right]}}
\end{align*}
Using the $t$ statistic above, a $\left(1-\alpha\right)100$% confidence interval for
$\beta_{0}$ is
\begin{align*}
b_{0}\pm t_{\alpha/2}\sqrt{s^2\left[\frac{1}{n}+\frac{\left(\bar{X}\right)^2}{\sum \left(X_i - \bar{X}\right)^2}\right]}\qquad\qquad\qquad(2.9)
\end{align*}
We can test the hypotheses
\begin{align*}
H_{0}: & \beta_{0}=\beta_{0}^{0}\\
H_{a}: & \beta_{0}\ne\beta_{0}^{0}
\end{align*}
where $\beta_{0}^{0}$ is the hypothesized value.
We can test this hypothesis with the $t$ test statistic assuming the null hypothesis is true: \begin{align*} t= & \frac{b_{0}-\beta_{0}^{0}}{\sqrt{s^2\left[\frac{1}{n}+\frac{\left(\bar{X}\right)^2}{\sum \left(X_i - \bar{X}\right)^2}\right]}}\qquad\qquad\qquad(2.10) \end{align*}
In Example 2.2.1, we showed how to do a confidence interval and hypothesis test for $\beta_1$. The R code also provided a confidence interval and hypothesis test for $\beta_0$. Note that the hypothesis test was testing the hypothesis $H_a:\beta_0=0$.
We can test this hypothesis with the $t$ test statistic assuming the null hypothesis is true: \begin{align*} t= & \frac{b_{0}-\beta_{0}^{0}}{\sqrt{s^2\left[\frac{1}{n}+\frac{\left(\bar{X}\right)^2}{\sum \left(X_i - \bar{X}\right)^2}\right]}}\qquad\qquad\qquad(2.10) \end{align*}
In Example 2.2.1, we showed how to do a confidence interval and hypothesis test for $\beta_1$. The R code also provided a confidence interval and hypothesis test for $\beta_0$. Note that the hypothesis test was testing the hypothesis $H_a:\beta_0=0$.