Fill in Blanks
Home
1.1 Bivariate Relationships
1.2 Probabilistic Models
1.3 Estimation of the Line
1.4 Properties of the Least Squares Estimators
1.5 Estimation of the Variance
2.1 The Normal Errors Model
2.2 Inferences for the Slope
2.3 Inferences for the Intercept
2.4 Correlation and Coefficient of Determination
2.5 Estimating the Mean Response
2.6 Predicting the Response
3.1 Residual Diagnostics
3.2 The Linearity Assumption
3.3 Homogeneity of Variance
3.4 Checking for Outliers
3.5 Correlated Error Terms
3.6 Normality of the Residuals
4.1 More Than One Predictor Variable
4.2 Estimating the Multiple Regression Model
4.3 A Primer on Matrices
4.4 The Regression Model in Matrix Terms
4.5 Least Squares and Inferences Using Matrices
4.6 ANOVA and Adjusted Coefficient of Determination
4.7 Estimation and Prediction of the Response
5.1 Multicollinearity and Its Effects
5.2 Adding a Predictor Variable
5.3 Outliers and Influential Cases
5.4 Residual Diagnostics
5.5 Remedial Measures
3.2 The Linearity Assumption
"You can observe a lot by just watching." - Yogi Berra
We can check the linearity assumption by plotting the residuals vs the predictor variable or plotting the residuals vs the fitted values.
We usually examine ascatterplot to determine if a linear relationship between $X$ and $Y$ is appropriate. There are times when the scatterplot makes it difficult to see if a nonlinear relationship exists. This may be the case if the observed $Y$ are close to the fitted line $\hat{Y}_i$. This usually means the slope is steep.
We usually examine a
In this example, we will consider the weights (in kg) and heights (in m) of 16 women ages 30-39. The dataset is from kaggle.
library(tidyverse)
#read in data from website
dat = read_csv("http://www.jpstats.org/Regression/data/Weight_Height.csv",)
#plot the data
ggplot(dat, aes(x=Weight, y=Height))+
geom_point()
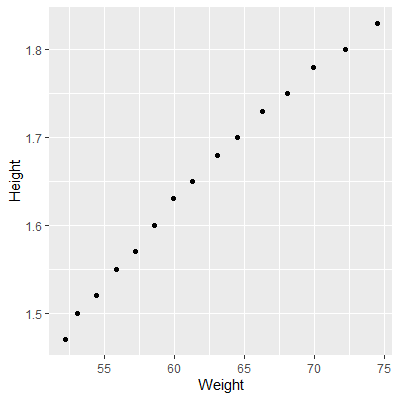
#fit the model
fit = lm(Weight~Height, data=dat)
#plot with regression line
ggplot(dat, aes(x=Weight, y=Height))+
geom_point()+
geom_smooth(method="lm", formula=y~x, se=F)
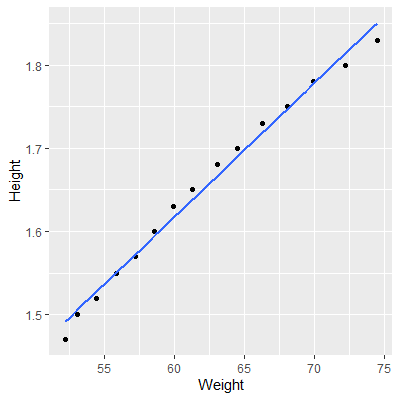
#make dataset with Weight, the fitted values, and residuals
dat2 = tibble(x = dat$Weight, yhat = fit$fitted.values, e = fit$residuals)
#plot x by residuals
ggplot(dat2, aes(x=x, y=e))+
geom_point()+
geom_hline(yintercept = 0, col="red")
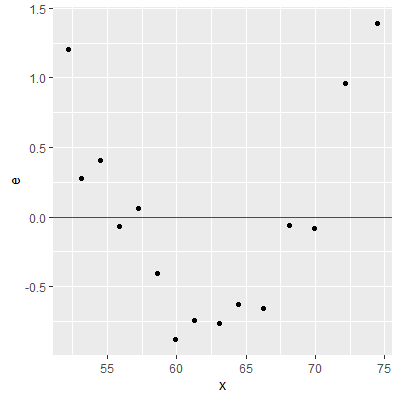
#plot fitted values by residuals
ggplot(dat2, aes(x=yhat, y=e))+
geom_point()+
geom_hline(yintercept = 0, col="red")
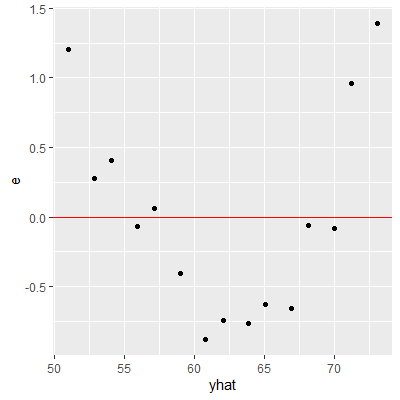
Plotting the residuals against $X$ will provide the same information as plotting the residuals against $\hat{Y}$ for the simple linear regression model.
When more predictor variables are considered (Chapter 4), then plotting against the $X$ variables and plotting against $\hat{Y}$ may provide different information. It is usually helpful to plot both in that case.
When more predictor variables are considered (Chapter 4), then plotting against the $X$ variables and plotting against $\hat{Y}$ may provide different information. It is usually helpful to plot both in that case.
When the linearity assumption does not hold (as seen in the residual plots), then a nonlinear model may be considered or a transformation on either $X$ or $Y$ can be attempted to make the relationship linear.
Transforming the response variable $Y$ may lead to issues with other assumptions such as the
constant variance assumption or the normality of $\varepsilon$ assumption.
If our only concern is the linearity assumption, then transforming $X$ will be the best option. This transformation may be a square root transformation $\sqrt{X}$, a log transformation $\log{X}$, or some power transformation $X^{p}$ were $p$ is some real number.
Sometimes a transformation of $X$ will not be enough to satisfy the linearity assumption. In that case, model (2.1)
If our only concern is the linearity assumption, then transforming $X$ will be the best option. This transformation may be a square root transformation $\sqrt{X}$, a log transformation $\log{X}$, or some power transformation $X^{p}$ were $p$ is some real number.
Sometimes a transformation of $X$ will not be enough to satisfy the linearity assumption. In that case, model (2.1)
\begin{align*}
Y_i=&\beta_0+\beta_1X_i+\varepsilon_i\\
\varepsilon_i\overset{iid}{\sim}& N\left(0,\sigma^2\right)\qquad\qquad\qquad\qquad(2.1)
\end{align*}
should be abandoned in favor of a nonlinear model.